Keynote Speakers
Juan-Pablo ORTEGA
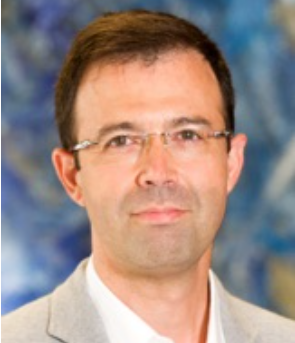
Juan-Pablo ORTEGA
Head, Division of Mathematical Sciences. Associate Chair (Faculty), School of Physical and Mathematical Sciences. Nanyang Technological University, Singapore
- Learning of Dynamic Processes -
Abstract: The last decade has seen the emergence of learning techniques that use the computational power of dynamical systems for information processing. Some of those paradigms are based on architectures that are partially randomly generated and require a relatively cheap training effort, which makes them ideal in many applications. The need for a mathematical understanding of the working principles underlying this approach, collectively known as Reservoir Computing, has led to the construction of new techniques that put together well-known results in systems theory and dynamics with others coming from approximation and statistical learning theory. This combination has allowed in recent times to elevate Reservoir Computing to the realm of provable machine learning paradigms and, as we will see in this talk, it also hints at various connections with kernel maps, structure-preserving algorithms, and physics-inspired learning.
References:
- Gonon, L., Grigoryeva, L., and Ortega, J.-P. [2022] Approximation bounds for random neural networks and reservoir systems. To appear in The Annals of Applied Probability. Paper
- Cuchiero, C., Gonon, L., Grigoryeva, L., Ortega, J.-P., and Teichmann, J. [2021] Expressive power of randomized signature. NeurIPS 2021. Paper
- Cuchiero, C., Gonon, L., Grigoryeva, L., Ortega, J.-P., and Teichmann, J. [2021] Discrete-time signatures and randomness in reservoir computing. IEEE Transactions on Neural Networks and Learning Systems, 33(11):6321-6330. Paper
- Gonon, L. and Ortega, J.-P. [2021] Fading memory echo state networks are universal. Neural Networks, 138, 10-13. Paper
- Gonon, L., Grigoryeva, L., and Ortega, J.-P. [2020] Risk bounds for reservoir computing. Journal of Machine Learning Research, 21(240), 1-61. Paper
- Gonon, L. and Ortega, J.-P. [2020] Reservoir computing universality with stochastic inputs. IEEE Transactions on Neural Networks and Learning Systems, 31(1), 100-112. Paper
- Grigoryeva, L. and Ortega, J.-P. [2019] Differentiable reservoir computing. Journal of Machine Learning Research, 20(179), 1-62. Paper
- Grigoryeva, L. and Ortega, J.-P. [2018] Echo state networks are universal. Neural Networks, 108, 495-508. Paper
- Grigoryeva, L. and Ortega, J.-P. [2018] Universal discrete-time reservoir computers with stochastic inputs and linear readouts using non-homogeneous state-affine systems. Journal of Machine Learning Research, 19(24), 1-40. Paper
Hervé SABOURIN
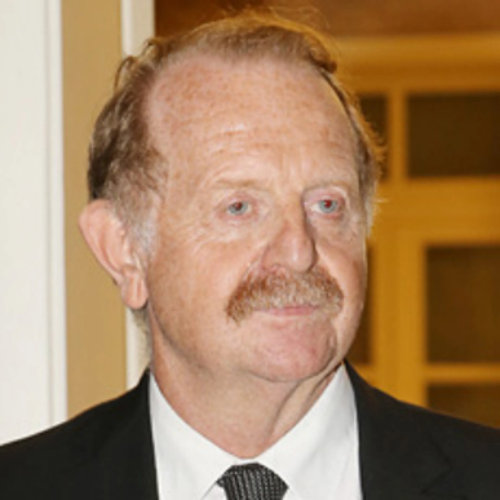
Hervé SABOURIN
Director for Strategic projects of the Réseau Figure® (network of 31 universities)
Former Regional Director of the A.U.F (Agence Universitaire de la Francophonie) for the Middle East
Former Vice-President of the University of Poitiers (France)
Title: Transverse Poisson Structures to adjoint orbits in a complex semi-simple Lie algebra
Abstract:
The notion of transverse Poisson structure has been introduced by Arthur Weinstein stating in his famous splitting theorem that any Poisson Manifold M is, in the neighbourhood of each point m, the product of a symplectic manifold, the symplectic leaf S at m, and a submanifold N which can be endowed with a structure of Poisson manifold of rank 0 at m. N is called a transverse slice at M of S. When M is the dual of a complex Lie algebra g equipped with its standard Lie-Poisson structure, we know that the symplectic leaf through x is the coadjoint G. x of the adjoint Lie group G of g. Moreover, there is a natural way to describe the transverse slice to the coadjoint orbit and, using a canonical system of linear coordinates (q1, ….., qk), it follows that the coefficients of the transverse Poisson structure are rational in (q1, ….., qk). Then, one can wonder for which cases that structure is polynomial. Nice answers have been given when g is semi-simple, taking advantage of the explicit machinery of semi-simple Lie algebras. One shows that a general adjoint orbit can be reduced to the case of a nilpotent orbit where the transverse Poisson structure can be expressed in terms of quasihomogeneous polynomials. In particular, in the case of the subregular nilpotent orbit the Poisson structure is given by a determinantal formula and is entirely determined by the singular variety of nilpotent elements of the slice.
References:
- Sabourin , H. , Sur la structure transverse à une orbite nilpotente adjointe, Canad.J.Math, 57, (4), 2005, p 750-770.
- Sabourin, H. , Orbites nilpotentes sphériques et représentations unipotentes associées : Le cas SL(n), Representation Theory, 9, (2005), p 468-506.
- Sabourin, H. , Mémoire d'HDR, Quelques aspects de la méthode des orbites en théorie de Lie, Décembre 2005
- Damianou, P. , Sabourin, H. , Vanhaecke, P. , Transverse Poisson structures to adjoint orbits in semi-simple Lie algebras, Pacific J. Math,232, 111-139, 2007.
- Sabourin, H., Damianou, P. , Vanhaecke, P., Transverse Poisson structures : the subregular and the minimal orbits, Differential Geometry and its Applications Proc. Conf. in Honour of Leonhard Euler, Olomouc, August 2007
- Sabourin, H., Damianou, P. , Vanhaecke, P., Nilpotent orbits in simple Lie algebras and their transverse Poisson structures, American Institute of Physics, Conf. Proc. series, Vol. 1023 (2008), pp 148-152.
Francis BACH
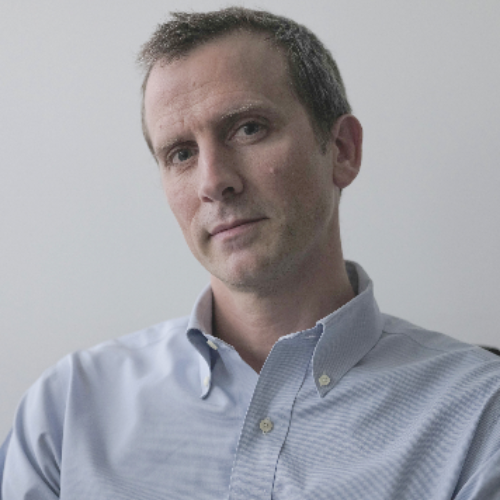
Francis BACH
Inria, Ecole Normale Supérieure
Title : Information Theory with Kernel Methods
Abstract: Estimating and computing entropies of probability distributions are key computational tasks throughout data science. In many situations, the underlying distributions are only known through the expectation of some feature vectors, which has led to a series of works within kernel methods. In this talk, I will explore the particular situation where the feature vector is a rank-one positive definite matrix, and show how the associated expectations (a covariance matrix) can be used with information divergences from quantum information theory to draw direct links with the classical notions of Shannon entropies.
References:
Francis Bach. Information Theory with Kernel Methods. To appear in IEEE Transactions in Information Theory, 2022. https://arxiv.org/pdf/2202.08545
Eva MIRANDA
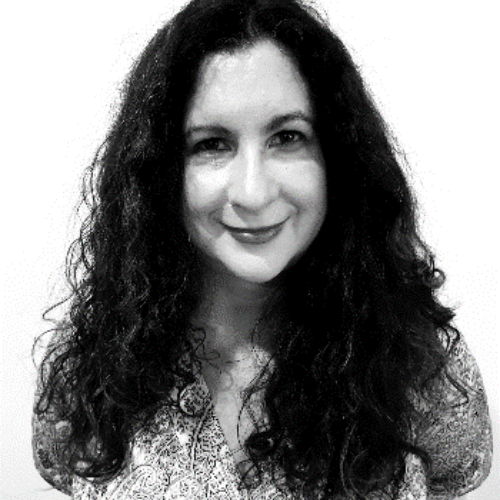
Eva MIRANDA
Universitat Politècnica de Catalunya and Centre de Recerca Matemàtica
Title: From Alan Turing to Contact geometry: towards a "Fluid computer”
Abstract: Abstract: Is hydrodynamics capable of performing computations? (Moore 1991). Can a mechanical system (including a fluid flow) simulate a universal Turing machine? (Tao, 2016).
Etnyre and Ghrist unveiled a mirror between contact geometry and fluid dynamics reflecting Reeb vector fields as Beltrami vector fields. With the aid of this mirror, we can answer in the positive the questions raised by Moore and Tao. This is a recent result that mixes up techniques from Alan Turing with modern Geometry (contact geometry) to construct a "Fluid computer" in dimension 3. This construction shows, in particular, the existence of undecidable fluid paths. I will also explain applications of this mirror to the detection of escape trajectories in Celestial
mechanics (for which I'll need to extend the mirror to a singular set-up). This mirror allows us to construct a tunnel connecting problems in Celestial mechanics and Fluid Dynamics.
References:
Robert Cardona, Eva Miranda, Daniel Peralta-Salas, and Francisco Presas, Constructing Turing complete Euler flows in dimension 3. Proc. Natl. Acad. Sci. USA 118 (2021), no. 19, Paper No. e2026818118, 9 pp.
- Etnyre, R. Ghrist, Contact topology and hydrodynamics: I. Beltrami fields and the Seifert conjecture. Nonlinearity 13, 441 (2000).
Eva Miranda, Cédric Oms and Daniel Peralta-Salas, On the singular Weinstein conjecture and the existence of escape orbits for b-Beltrami fields. Commun. Contemp. Math. 24 (2022), no. 7, Paper No. 2150076, 25 pp.
- Tao, Finite time blowup for an averaged three-dimensional Navier–Stokes equation. J. Am. Math. Soc. 29, 601–674 (2016).
Alan Turing, On Computable Numbers, with an Application to the Entscheidungsproblem. Proceedings of the London Mathematical Society. Wiley. s2-42 (1): 230–265. doi:10.1112/plms/s2-42.1.230. ISSN 0024-6115., (1937).
Diarra FALL
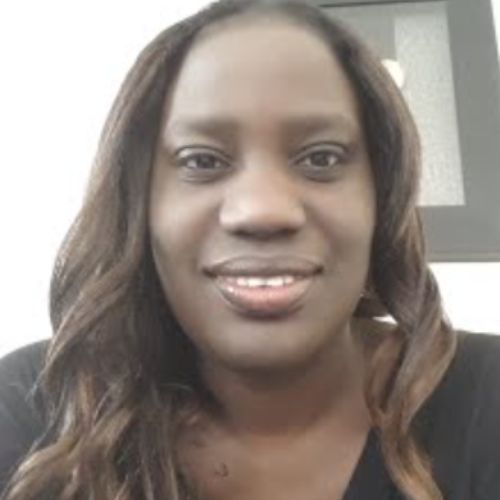
Diarra FALL
Institut Denis Poisson, UMR CNRS, Université d'Orléans & Université de Tours, France.
Title: Statistics Methods for Medical Image Processing and Reconstruction
Abstract :
In this talk we will see how statistical methods, from the simplest to the most advanced ones, can be used to address various problems in medical image processing and reconstruction for different imaging modalities. Image reconstruction allows to obtain the images in question, while image processing (on the already reconstructed images) aims at extracting some information of interest. We will review several statistical methods (manely Bayesian) to address various problems of this type.
Keywords: Image processing, image reconstruction, Statistics, frequentist, Bayesian, Parametrics, Nonparametrics.
References
- D. Fall, N. Dobigeon, P. Auzou, "A Bayesian Estimation Formulation to Voxel-Based Lesion Symptom Mapping", Proc. European Signal Processing Conf. (EUSIPCO), Belgrade, Serbia, Sept. 2022.
- D. Fall, "Bayesian Nonparametrics and Biostatistics: the case of PET Imaging", International Journal of Biostatistics, 2019.
- D. Fall, E. Lavau and P. Auzou, "Voxel-Based Lesion-Symptom Mapping: a Nonparametric Bayesian Approach", Proc. IEEE International Conference on Acoustics, Speech and Signl Processing (ICASSP) 2018.
Bernd STURMFELS
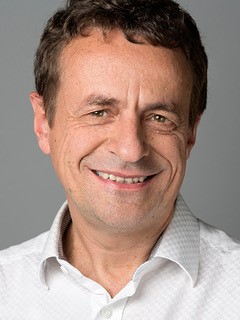
Bernd STURMFELS
MPI-MiS Leipzig, Germany
- Algebraic Statistics and Gibbs Manifolds -
Abstract: Gibbs manifolds are images of affine spaces of symmetric matrices under the exponential map. They arise in applications such as optimization, statistics and quantum physics, where they extend the ubiquitous role of toric geometry. The Gibbs variety is the zero locus of all polynomials that vanish on the Gibbs manifold. This lecture gives an introduction to these objects from the perspective of Algebraic Statistics.
References:
- Pavlov, B.Sturmfels and S.Telen: Gibbs manifolds, arXiv:2211.15490
- Sturmfels, S.Telen, F-X.Vialard and M.von Renesse: Toric geometry of entropic regularization, arXiv:2202.01571
- Sullivant: Algebraic statistics. Graduate Studies in Mathematics, 194, American Mathematical Society, Providence, RI, 2018
- Huh and B.Sturmfels: Likelihood geometry, in Combinatorial Algebraic Geometry (eds. Aldo Conca et al.), Lecture Notes in Mathematics 2108, Springer (2014) 63-117.
- Geiger, C.Meek and B.Sturmfels: On the toric algebra of graphical models, Annals of Statistics 34 (2006) 1463-1492